Experimenting with Electric Vehicles in Kigali
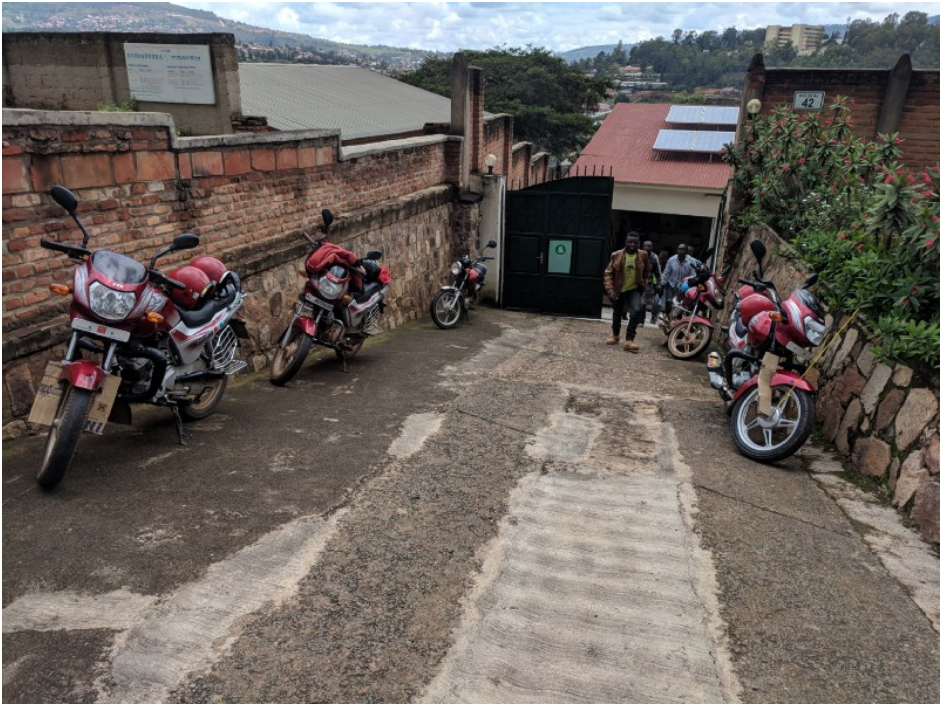
Imagine…
You are a farmer in rural Mozambique. You have a cough. You walk five miles to your nearest health clinic to have a tuberculosis test. Two weeks later you go back to find out the results, only to be told the sample didn’t make it to the laboratory. Could drones make this last mile dependable and efficient?
You are an adolescent woman in a slum in Malawi, vulnerable to sexual violence. Emergency contraception might just provide you with a lifeline, but the only access point free from stigma is a contraceptive vending machine that is out of stock. Could Internet of Things-enabled vending machines prompt for stock fulfilment when they run out to make this resource more reliable?
Frontier Technology Livestreaming (FTL) is a program from UKAid, designed to explore the application of frontier technologies like drones and Internet of Things (IoT) to solve humanity’s biggest, global development challenges using Lean Impact methods. We keep to task by:
- Maintaining proximity to the problem i.e. don’t be a hammer looking for a nail.
- Using a short amount of time and a small amount of money to create catalytic impact. i.e. build to learn rather than build to implement.
- Giving our teams space to pivot i.e. don’t sink time/money into things that aren’t working.
To improve air quality in Kigali, the capital of Rwanda, we’re working with Ampersand to switch the city’s taxi drivers from petrol to electric vehicles.
The motorbike taxi (or ‘moto’) is by far the most common vehicle in Kigali. Across East Africa these motos cover enough distance to travel to the sun and back, twice a day.
Using advances in electric vehicle technology, we’re exploring how we might develop a greener alternative to the classic petrol moto, that – if it works – can be scaled across East Africa.
Building Something People Want
To bridge the gap between high-functioning tech and an ambitious rollout, we’ve been working with our technology partner Ampersand to rapidly test and learn about the end user (taxi drivers) and the environment their electric motos will operate in.
This testing starts with the value hypothesis within our idea: is it something people actually want?
We convened a session giving five taxi drivers the chance to come face-to-face with five different taxi prototypes and ride one, followed by a secret ballot to decide which they liked best. To our surprise, the one they unanimously liked most was the one most similar to the one they already used; in the eyes of our end user, familiarity trumped our attempts to make the bike more comfortable, functional or futuristic.
The Ampersand prototype motorbike.
Finding Our Benchmark to Hack Affordability
We’ve also used hacks to save money and crucial months of time in testing our growth hypothesis: taxi drivers will pay for the bike and it will scale.
To work out whether our bike could compete with petrol for affordability, we had to know how much our customer really spends on fuel. Tough when the drivers themselves don’t have a clue – the fuel gauges on their bikes had stopped working long ago. We discovered that drivers mostly just pat their pocket, and put another buck of gas in the tank when they’ve earned $4 or $4.50.
We recruited some drivers, gave each a fanny pack (or bum bag) containing a smartphone utilizing Strava (a running app). At the start of each working day, we drained their fuel tank and put in exactly five litres (1.3 gal) of fuel. At the end of the day we drained the tank, measured how much petrol was left, and compared with GPS data from Strava. After a couple of weeks, we realized the drivers covered, on average, 188 km (117 miles) in a day, and at today’s prices spent $5.65 on fuel. This was our benchmark to beat.
We also conducted fifty one-hour interviews with taxi drivers, diving deep into how much they earn. We discovered a typical taxi driver makes a mere $1 a day of profit. So even a marginal decrease in their fuel costs, means a potentially significant percentage increase in how much they take home.
By using a GPS-based running app and filling up bikes with a fixed amount of fuel, we were able to quickly calculate the average daily profit for the typical Kigali taxi driver. Share on XHacking our ‘Affordability Test’
Testing the per charge range (km) of an electric vehicle typically means using an expensive dynamometer ordered from overseas, which in our case was delayed for months due to supplier issues. A dynamometer is, in layman’s terms, a test-stand that can be programmed to simulate different conditions without leaving the factory.
Using Google Earth Pro (our hack for a dynamometer), we played around until we came up with a three km (1.9 miles), real-world circuit around Kigali we believed to be representative of the city. Then we got our prototype bike and rode around the route again and again, collecting data on the vehicle’s range on one charge, and the variables that might affect it. We quickly and cheaply went 80% of the way to confirming our hypothesis that our electric moto would outperform petrol bikes on efficiency and performance on the streets of Kigali.
Our Next Experiments? Testing the Sales Model
Our next set of experiments will delve further into the sales model of the bike itself. Lease-to-own models are the most popular in Kigali (and we already know that taxi drivers like familiarity), but could we try something different? For example, might renting be preferable to lease-to-own, if it was cheaper (and covered maintenance)?
We will look to validate answers to these questions through rough and ready financial modeling (emphasizing speed and 80% accuracy over perfection) and more workshops with local taxi drivers.
Lean Testing for Impact
Finally, our impact hypothesis rests on two pillars. One is that our electric bikes reduce air pollution, which saves lives. In addition, more take home income for drivers also means a segment of Kigali’s lower-income population has more money – enabling access to better healthcare, nutrition, and education. We will carry out independent assessments to validate these assumptions further down the line.
Had we simply drawn up what we thought was the “best” solution on a whiteboard (based on our own assumptions and experience), built hundreds and rolled them via an implementation program in a traditional, waterfall management style, we would have wasted a lot of time and money in pushing through tech not optimized for its local environment.
By focusing our work around validating assumptions against all three hypotheses, and applying the Lean Impact methodology, we believe we’re giving this tech a real chance for reaching scale and making a meaningful, enduring impact on people’s lives.
Had we simply implemented what we believed to be the best solution, built hundreds & rolled them out via traditional ‘waterfall’ management, we would have wasted a lot of time & money pushing through tech not optimized for… Share on XStay tuned for more…
Thank you to Lea Simpson and Asad Rahman from Brink, and Josh Whale from Ampersand, for contributing this piece. In the coming weeks, they’ll share more posts looking at some of Frontier Technology Livestreaming’s drones, IoT, and other pilots. Give us a shout out on Twitter @leanimpact and let us know what you thought about this Lean Impact piece!